5 Steps To Collect High-Quality Data
There’s no question that in statistics, you need to ensure that the data that you collect has good quality. However, unlike what you may think, this isn’t always an easy task. The truth is that a company may experience quality issues when integrating data sets from various applications or departments or when entering data manually. So, we decided to share with you the steps you need to proceed when you want to collect high-quality data.
5 Steps To Collect High-Quality Data
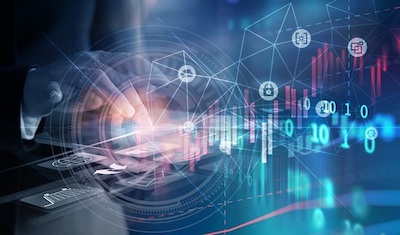
#1: Data Governance Plan:
When you are looking to collect high-quality data, you need to ensure that you begin with a data governance plan. Simply put, this plan shouldn’t only talk about ownership but also about classification, sharing, and sensitivity levels. But above all, it’s important that it follows in detail with procedural details that outline your data quality goals.
So, you need to ensure that it has the details of all the personnel involved in the process and each of their roles and more importantly a process to resolve/work through issues.
Ultimately, you can see data governance as the process of ensuring that there are data curators who are looking at the information being ingested into the organization and that there are processes in place to keep that data internally consistent, making it easier for consumers of that data to get access to it in the forms that they need.
Learn more about the F distribution.
#2: Data Quality Guidance:
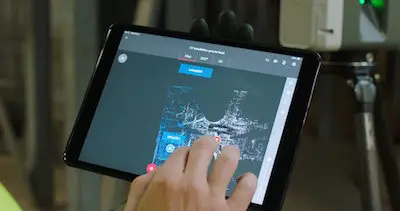
When you collect high-quality data, you know you need to separate good data from bad data. This means you need to have a clear guide to use.
Overall speaking, you will need to calibrate your automated data quality system with this information, so you need to have it laid out beforehand. Notice that this step also includes the validation of the data before it can be further processed. This ensures that data meets minimal standards.
This is how you make an histogram.
#3: Data Cleansing Process:
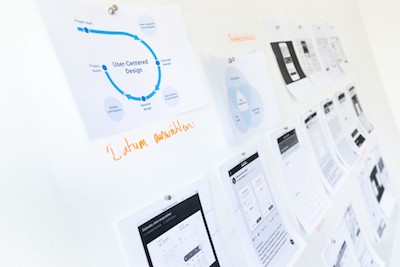
While you may have a good process in place to set apart good data from bad data, you still need to use a data cleansing process to look for flaws in your datasets.
You need to make sure that you provide guidance on what to do with specific forms of bad data and identifying what’s critical and common across all organizational data silos.
One of the things to keep in mind is that implementing data cleansing manually is cumbersome as the business shifts, strategies dictate the change in data and the underlying process.
#4: Clear Data Lineage:
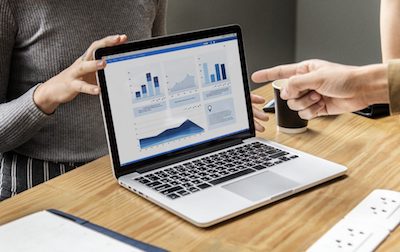
When you want to collect high-quality data, you know that this data comes from different departments and digital systems. So, it’s imperative that you have a clear understanding of data lineage. This means knowing how an attribute is transformed from system to system interactions and provide the ability to build trust and confidence.
Simply put, data lineage is metadata that indicates where the data was from, how it has been transformed over time, and who, ultimately, is responsible for that data.
Discover what sampling variability is and why it is important.
#5: Data Catalog And Documentation:
The last step of how to collect high-quality data is related to data catalog and documentation.
Improving data quality is a long-term process that you can streamline using both anticipations and past findings.
So, when you document every problem that is detected and associated data quality score to the data catalog, you reduce the risk of mistake repetition and solidify your data quality enhancement regime with time.