When you are learning statistics, then you probably already know that there aren’t many statistical methods that you can use with ordinal variables. However, you have options. But it’s important to understand that some options are better than others.
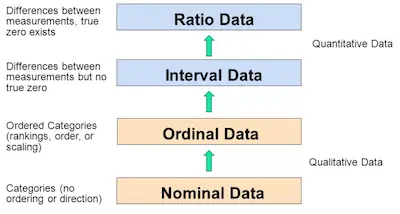
So, whenever you have an ordinal dependent variable, then check out the options that you have.
5 Ways to Analyze Ordinal Variables
#1: Treat Ordinal Variables As Nominal:
While ordinal variables are fundamentally categorical, then one of the options that you have is to simply ignore the order in the categories of variables and treat is as nominal.
The truth is that this can make sense for a wide range of variables and the biggest advantage is the fact that by adopting this approach, you won’t be violating any assumptions.
Looking to determine the z score?
#2: Treat Ordinal Variables As Numeric:
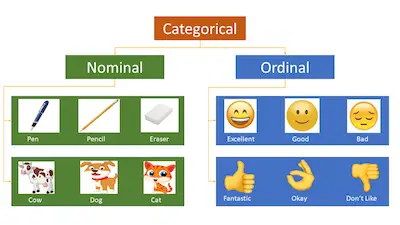
One of the things that happen a lot of times id that ordering categories is central to the research question. So, what many data analysts do is exactly the opposite: they simply ignore the fact that the ordinal variable isn’t numerical and treat the numerals that designate each category as actual numbers.
A big disadvantage of using this approach is the fact that you need to assume that the distance between each set of subsequent categories is equal. And that can be very difficult to justify.
Use our simple a score calculator.
#3: Non-Parametric Tests:
While you may think that these tests are only useful for numerical data that are non-normal or have outliers, the reality is that you can also use these tests for ordinal variables. And this especially true and should be considered especially when you have more than just a few ordered categories.
Some of the non-parametric tests that are often used include the Friedman, the Wilcoxon-Mann-Whitney, the Spearman correlation, and the Kruskal-Wallis.
However, there is a big catch when you decide to use these tests. The reality is that they are very basic. After all, you won’t even be able to include covariates or interactions among two independent variables.
#4: Ordinal Logistic And Probit Regression:
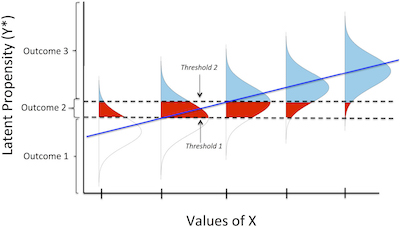
One of the tests that tend to be very used for ordinal variables is the logistic (or probit) regression.
One of the things that you need to keep in mind about this test is the fact that you can specify the logit link function in different ways so that you can maintain the order in the dependent variable. The most commonly available in software is the cumulative link function, which allows you to measure the effect of predictors on the odds of moving into any next-highest-ordered category.
Notice that these models are very complex and they shouldn’t be used by a beginner. In addition, you should also keep in mind that these models have their own assumptions and they take time to learn how to interpret them.
Looking for an easy z-score calculator to use?
#5: Rank Transformations:
The last model that you can use for ordinal variables is to simply rank transformations. Ultimately, this is the combination between the simplicity of the rank-based non-parametrics and the advantages of ordinal logistic regression.
The main idea of this model is to transform each ordinal outcome score into the rank of that score and run your regression, two-way ANOVA, or other model on those ranks.
Notice that all the results that you get need to be interpreted in terms of the ranks.