Generative and Analytical Models for Data Analysis
When you think about data, it is important that you keep in mind that there are two different approaches that you can adopt: the generative and the analytical approach.
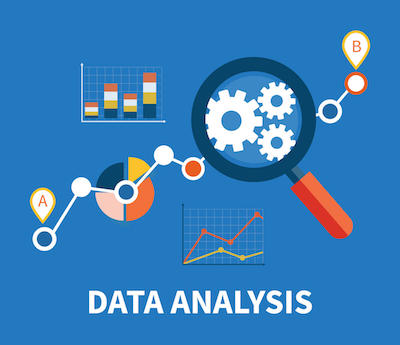
So, let’s take a look at each one of these models for data analysis.
Learn everything you need to know about statistics.
Generative Model For Data Analysis
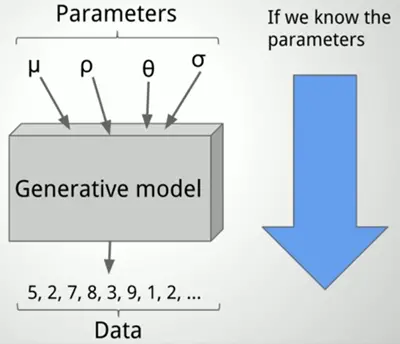
Simply put, when you use the generative model for data analysis, the process will focus on the process by which the analysis is created. This means that you need to develop an understanding of the decisions that you make from one step to the other so that you can recreate or reconstruct a data analysis.
One of the things that you need to keep in mind about this model is the fact that it tends to take place inside the data analyst’s head which means that it can’t b observed directly. So, when you need to take measurements, you will need to ask the analyst directly. However, the main problem is that this is subjected to a wide range of measurement errors. Notice that on some occasions, you may have access to partial information when the analyst writes down the thinking process through a series of reports or if a team is involved and there is a record of communication about the process.
Discover the different types of correlation.
This model tends to be quite useful for understanding the “biological process”, i.e. the underlying mechanisms for how data analyses are created, sometimes referred to as “statistical thinking”.
Analytic Model For Data Analysis
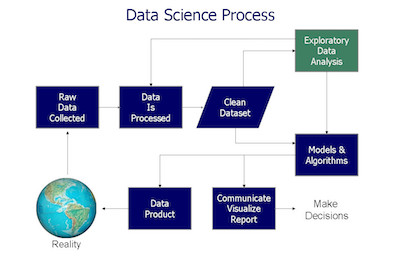
With this approach, you will ignore the underlying processes that serve to generate the data analysis and you will focus on the observable outputs of the analysis. These outputs may be an R markdown document, a PDF report, or even a slide deck.
The main advantage of using this approach is that the analytic outputs are real and can be directly observed. However, it’s worth noting that the elements placed in the report are the cumulative result of all the decisions made through the course of a data analysis.
Many people tend to refer to the analytical model for data analysis as the physician approach since it basically mirrors the problem that a physician confronts.
Understanding predictive analytics.
What Is Still Missing?
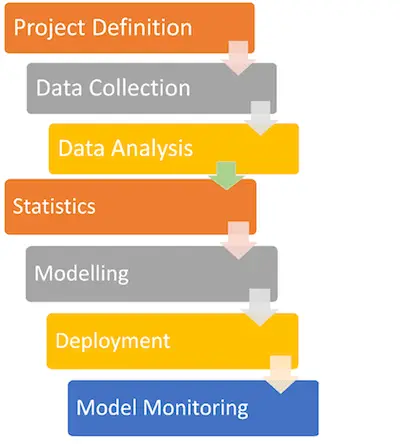
After analyzing both models – the generative and the analytical models for data analysis, it is worth to state that we believe that something is still missing.
The reality is that when you are gathering new data, you need to think about the answers that you’re trying to get. This ensures that you need to achieve a balance between matching the principles of both the analyst and the audience. So, summing up, for both the generative model and the analytical model of data analysis, the missing ingredient is a clear definition of what makes a data analysis successful. The other side of that coin, of course, is knowing when a data analysis has failed.
Check out the ultimate guide to descriptive statistics.
While the analytical approach is useful because it allows you to separate the analysis from the analyst and to categorize analyses according to their observed features, the categorization is unordered unless we have some notion of success.
On the other hand, the generative approach is useful because it reveals potential targets of intervention, especially from a teaching perspective, in order to improve data analysis. However, without a concrete definition of success, you don’t have a target to strive for and you do not know how to intervene in order to make genuine improvement.