The Difference Between Model Assumptions, Inference Assumptions, And Data Issues
One of the things that you may have never noticed before is that the list of assumptions for linear regression that you find on textbooks, lecture notes, or websites may be different. But why does this happen?
Discover the best statistics calculators online.
The reality is that authors use different terminology. So, while they are using the same assumptions, they look different. However, it’s important to keep in mind that sometimes they’re including not only model assumptions but inference assumptions and data issues. While they are all important, it’s even more important to understand the role of each can help you understand what applies in your situation.
Model Assumptions
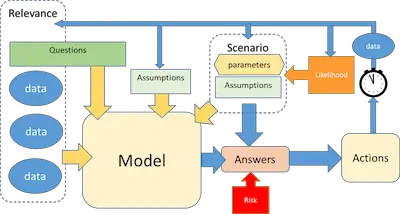
Simply put, the model assumptions are all about the specification and performance of the model for estimating the parameters well.
1. The errors are independent of each other
2. The errors are normally distributed
3. The errors have a mean of 0 at all values of X
4. The errors have constant variance
5. All X are fixed and are measured without error
6. The model is linear in the parameters
7. The predictors and response are specified correctly
8. There is a single source of unmeasured random variance
Learn more about the t-test and the f-test.
Notice that not all these model assumptions will be stated explicitly not to mention that you can’t check them all. Ultimately, you need to make sure that you included all the “correct” predictors. Nevertheless, you shouldn’t skip the step of checking what you can. And for those you can’t, take the time to think about how likely they are in your study and report that you’re making those assumptions.
Assumptions About Inference
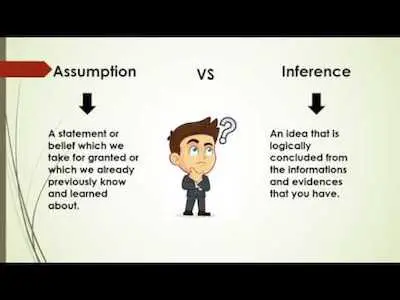
Sometimes the assumption is not really about the model, but about the types of conclusions or interpretations that you can make about the results.
These assumptions allow the model to be useful in answering specific research questions based on the research design. They’re not about how well the model estimates parameters.
Check out the analysis of variance explained.
As you know, studies are designed to answer specific research questions. And they can only do that if these inferential assumptions hold. But if they don’t, it doesn’t mean the model estimates are wrong, biased, or inefficient. It simply means you have to be careful about the conclusions you draw from your results. Sometimes this is a huge problem.
But these assumptions don’t apply if they’re for designs you’re not using or inferences you’re not trying to make. This is a situation when reading a statistics book that is written for a different field of application can really be confusing. They focus on the types of designs and inferences that are common in that field.
It’s hard to list out these assumptions because they depend on the types of designs that are possible given ethics and logistics and the types of research questions. But here are a few examples:
1. ANCOVA assumes the covariate and the IV are uncorrelated and do not interact.
2. The predictors in a regression model are endogenous.
3. The sample is representative of the population of interest.
Data Issues That Are Often Mistaken For Assumptions
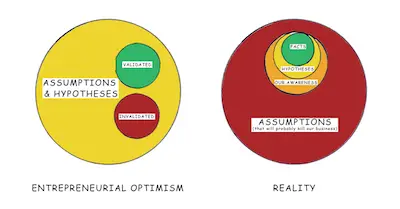
One of the things that tend to occur to many people is that their list of assumptions includes data issues that are a little different. However, they are still important. After all, they affect how you interpret the results as well as they impact how well the model performs.
What is a partial correlation?
When a model assumption fails, you can sometimes solve it by using a different type of model. Data issues generally stay around. That’s a big difference in practice.
Here are a few examples of common data issues:
1. Small Samples
2. Outliers
3. Multicollinearity
4. Missing Data
5. Truncation and Censoring
6. Excess Zeros