In statistics, you probably never even thought that there are some rules that you need to follow in what concerns rounding numbers.
The reality is that you need o deal with very large decimals and you may need to round them. So, what are the rules for rounding numbers in statistics? Should you simply follow the regular methods you learned in math classes?
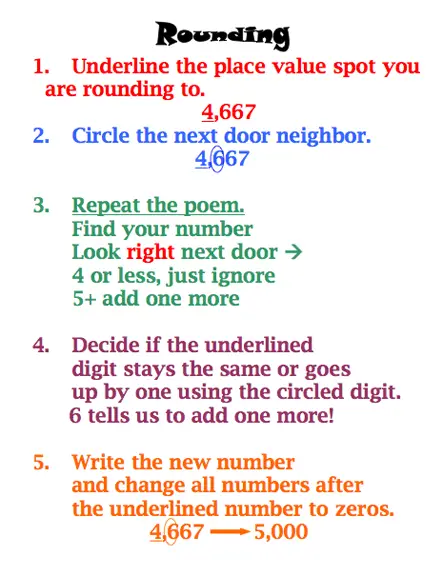
Before we get into details, it’s important that you are well aware of concepts such as digit, an even number, an odd number, decimal format versus decimal place, and particularly, and what is a significant digit. It is especially important to keep in mind that there is a very big difference between the decimal place and a significant digit.
For example, the numbers 0.00036, 36, and 36,000,000 all have 2 significant digits – 3 and 6 – but different decimal places.
Lear more about rounding numbers.
Rules For Rounding In Statistics
Step #1: Determine the Number of Significant Digits to Save
The number of significant digits to save is suggested by the precision of the measuring instrument for individual numbers and variability observed in a series of numbers.
The precision of a measurement refers to its repeatability, whereas accuracy relates to how close the measurement is to the truth. Standard deviation is an expression of the inherent variation of the numbers. In contrast, numbers such as regression coefficients are generally accompanied by their standard error, as the expression of the precision of those derived estimates. These measures of precision are the key to determining the number of significant digits to save.
For convenience, we will describe significant digits in terms of the mean and standard deviation. For cases in which the standard error is used, just translate ‘‘standard deviation’’ into ‘‘standard error.’’
Make sure to check out this rounding calculator.
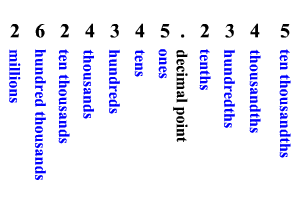
The place of the first significant digit of the standard deviation is found, and the mean or proportion is rounded to that place. The same place is saved in confidence limits. The standard deviation generally will be expressed to 1 additional place. Here are some examples:
#1: The mean age is 72.17986 and the standard deviation is 9.364132:
Nine is the first significant figure of the standard deviation and is in the ones place. Thus, we will keep 2 significant digits in the mean and 2 in the standard deviation: 72 and 9.4.
#2: The mean cost is $72,347.23 and the standard deviation is $23,994.06:
The 2 in the 10-thousands place is the first significant digit in the standard deviation. Thus, the mean cost would be expressed to 1 significant digit, and the standard deviation to 2 significant digits: $70,000 and $24,000.
Looking for a good rounding calculator to help you out?
Step #2: Look for Exceptions:
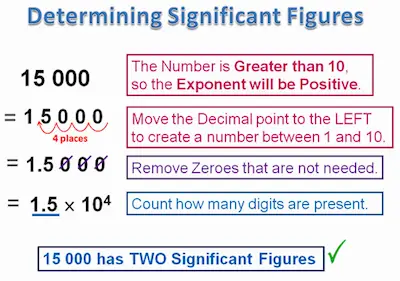
There are some exceptions that need to be considered. These include:
- If the first significant digit of the standard deviation is 1, then 1 additional significant digit in the mean or proportion and standard deviation may be saved.
- For percentages between 0% and 10% or between 90% and 100%, keep at least 2 significant digits.
- Within a single table, consistency in saving digits may be desirable, so all numbers may be rounded to the place indicated by the majority of the numbers.
Step #3: Round the Numbers:
Round the number by removing digits from its right side that falsely suggest a high degree of precision. This is how it’s done:
- If the digit in the first place beyond (to the right of) the significant digit to be rounded is > 5, add 1 to the right-most digit to be retained and drop all other digits to its right. This is called rounding up. Thus, 2.77 to 2 significant figures would be rounded to 2.8. Similarly, 1479.336 to 2 significant figures would be rounded to 1500 and 0.000649376 would be rounded to 0.00065.
- If the digit in the first place beyond the significant digit to be rounded is<5, simply drop it and all other digits to its right. This is called rounding down. Thus, 3.44 to 2 significant figures would be rounded to 3.4. Similarly, 98,432.19 would be rounded to 98,000 and 0.00013175 would be rounded to 0.00013.
- If the digit in the first place beyond the digit to be rounded is exactly 5, add 1 to the rightmost digit to be retained if the last significant digit is odd (ie, 1, 3, 5, 7, or 9), and leave the digit to be rounded as is if it is even (ie, 0, 2, 4, 6, or 8). This rule results in the rightmost significant digit always being an even number.5 Thus, 9.450000 to 2 significant figures would be rounded to 9.4, but 9.750000 would be rounded to 9.8.