When you are learning statistics and studying simple models, you are probably not aware that missing data is something very common in statistics. The reality is that date from experiments, surveys, and other sources are often missing some data.
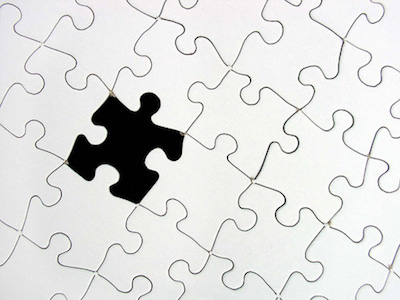
One of the most important things to keep in mind about missing data in statistics is that the impact of this missing data on the results depends on the mechanism that caused the data to be missing.
Looking for the best statistics calculators?
Data Are Missing For Many Reasons
- Subjects in longitudinal studies tend to drop out even before the study is complete. The reason is that they may have either died, moved to another area, or they simply don’t see a reason to participate.
- In what concerns surveys, these usually suffer from missing data when participants skip a question, don’t know, or don’t want to answer.
- In the case of experimental studies, missing data occurs when a researcher is unable to collect an observation. The researcher may become sick, the equipment may fail, bad weather conditions may prevent observation in field experiments, among others.
Discover how to interpret the F test.
Why Missing Data Is Important In Statistics
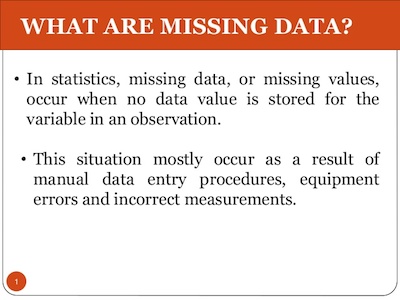
Missing data is a very important problem in statistics since most statistical procedures require a value for each variable. Ultimately, when a data set is incomplete, the data analyst needs to decide how to deal with it.
In most cases, researchers usually tend to use complete case analysis (also called listwise deletion). This means that they will be analyzing only the cases with complete data. Individuals with data missing on any variables are dropped from the analysis.
While this is a simple and easy to use approach, it has limitations. The most important limitation, in our opinion, is the fact that it can substantially lower the sample size, leading to a severe lack of power. This is especially true if there are many variables involved in the analysis, each with data missing for a few cases. Besides, it can also lead to biased results, depending on why the data are missing.
Learn why adding values on a scale can lead to measurement error.
Missing Data Mechanisms
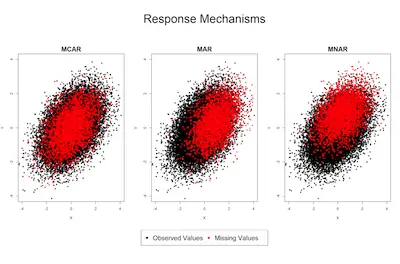
As we already mentioned above, the effects on your model will depend on the missing data mechanism that you decide to use.
Overall speaking, these mechanisms can be divided into 4 classes that are based on the relationship between the missing data mechanism and the missing and observed values.
These 3 designs look like repeated measures.
#1: Missing Completely At Random (MCAR):
MCAR means that the missing data mechanism is unrelated to the values of any variables, whether missing or observed.
Data that are missing because a researcher dropped the test tubes or survey participants accidentally skipped questions are likely to be MCAR. Unfortunately, most missing data are not MCAR.
#2: Non-Ignorable (NI):
NI means that the missing data mechanism is related to the missing values.
It commonly occurs when people do not want to reveal something very personal or unpopular about themselves. For example, if individuals with higher incomes are less likely to reveal them on a survey than are individuals with lower incomes, the missing data mechanism for income is non-ignorable. Whether income is missing or observed is related to its value.
#3: Missing At Random (MAR):
MAR requires that the cause of the missing data is unrelated to the missing values but may be related to the observed values of other variables.
MAR means that the missing values are related to observed values on other variables. As an example of CD missing data, missing income data may be unrelated to the actual income values but are related to education. Perhaps people with more education are less likely to reveal their income than those with less education.