Rules of thumb tend to b used sometimes in statistics. However, it is also important to keep in mind that if there is a rule of thumb, it also means that it may be misleading, misinterpreted, or simply wrong.
Discover the best online stats calculators.
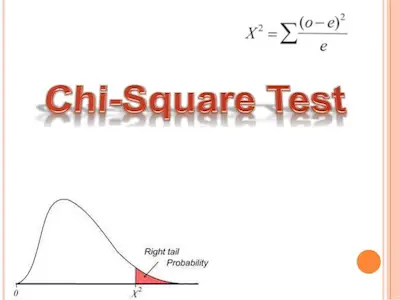
One of the rules of thumb that keeps getting distorted along the way regards the Chi-square test. You probably already heard that “The Chi-Square test is invalid if we have fewer than 5 observations in a cell”. However, this statement is not even accurate. If you are trying to say something similar to this, then you need to say that it’s the expected count that needs to be >5 per cell and not the observed in each cell.
Remembering The Chi-Square Test
As you probably already know, the Chi-square statistic follows a chi-square distribution asymptotically with df=n-1. This means that you can use the chi-square distribution to calculate an accurate p-value only for large samples. When you are working with small samples, it doesn’t work.
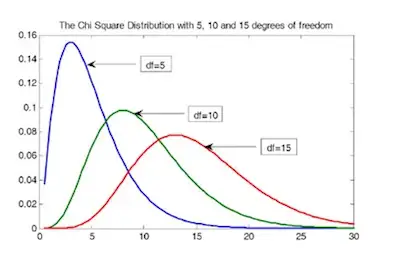
The Size Of The Sample
Now, you’re probably wondering about how large the sample needs to be. We can then state that it needs to be large enough that the expected value for each cell is at least 5.
Understanding the The Chi-Square Goodness Of Fit Test.
The expected values come from the total sample size and the corresponding total frequencies of each row and column. So, if any row or column totals in your contingency table are small, or together are relatively small, you’ll have an expected value that’s too low.
Just take a look at the table below, which shows observed counts between two categorical variables, A and B. The observed counts are the actual data. You can see that out of a total sample size of 48, 28 are in the B1 category and 20 are in the B2 category.
Likewise, 33 are in the A1 category and 15 are in the A2 category. Inside the box are the individual cells, which give the counts for each combination of the two A categories and two B categories.
Take a look at a reliable tool for Chi Square test online.
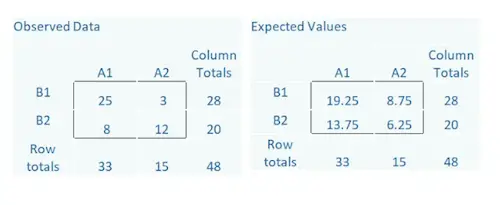
The Expected counts come from the row totals, column totals, and the overall total, 48.
For example, in the A2, B1 cell, we expect a count of 8.75. It is an easy calculation: (Row Total * Column Total)/Total. So (28*15)/48.
The more different the observed and expected counts are from each other, the larger the chi-square statistic.
Notice in the Observed Data there is a cell with a count of 3. But the expected counts are all >5. If the expected counts are less than 5 then a different test should be used such as the Fisher’s Exact Test.
Check out these 5 steps to calculate sample size.
Is It 5 The Real Minimum?
The truth is that other authors have suggested guidelines as well:
- All expected counts should be 10 or greater. If < 10, but >=5, Yates’ Correction for continuity should be applied.
- Fisher’s Exact and Yates Correction are too conservative and propose alternative tests depending on the study design.
- For tables larger than 2 x 2 “No more than 20% of the expected counts should be less than 5 and all individual expected counts should be greater or equal to 1. Some expected counts can be <5, provided none <1, and 80% of the expected counts should be equal to or greater than 5.
- The Minitab manual criteria are: If either variable has only 2 or 3 categories, then either:
— all cells must have expected counts of at least 3 or
— all cells must have expected counts of at least 2 and 50% or fewer have expected counts below 5
If both variables have 4 to 6 levels then either:
— all cells have expected counts of at least 2, or
— all cells have expected counts of at least 1 and 50% or fewer cells have expected counts of < 5.